Neural Radiance Fields (NeRF) is a recent implicit representation formulation of the volume that can generate novel views of complex 3D scenes, based on a partial set of 2D images. It is trained to maximize the rendering accuracy of the input images, optimizing the radiance field in terms of volumetric color and density. NeRF has revolutionized the field of implicit 3D representations, mainly due to a differentiable volumetric rendering formulation that enables high-quality rendering and geometry reconstruction.
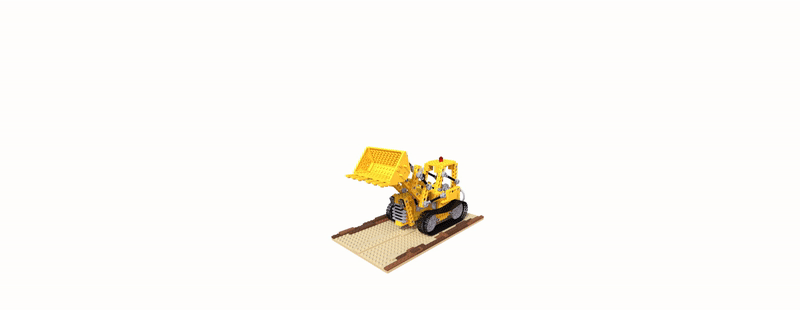

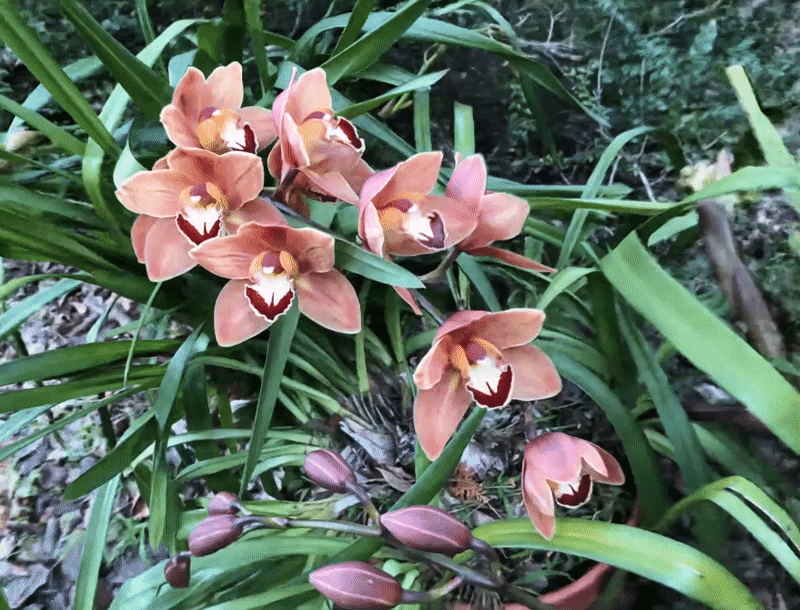
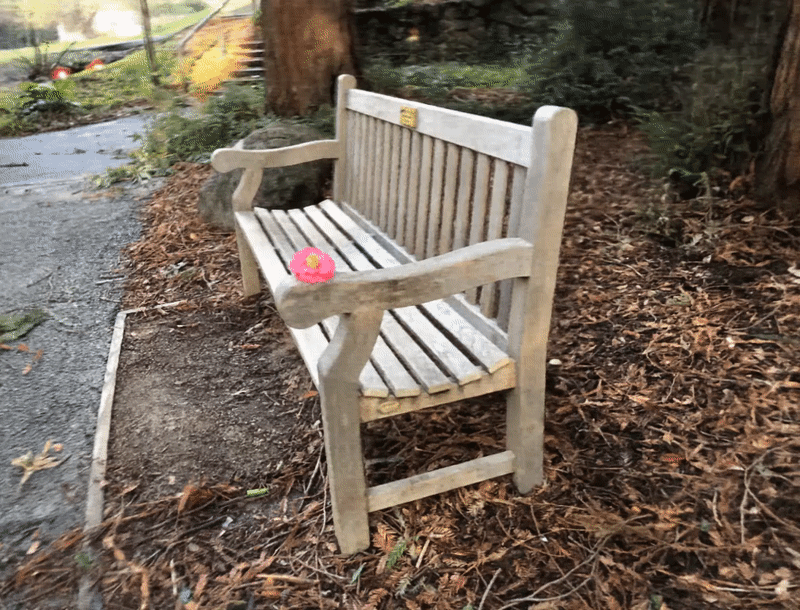
Even if initially developed for novel view synthesis, NeRF has shown incredible potentialities in terms of 3D reconstruction starting from RGB images. Traditional methods like Structure-from-Motion and Multi-view Stereo can only return a discrete representation (point clouds, mesh, voxel grids), struggling on texture-less regions. Implementing a new formulation of the volumetric density through the use of Signed Distance Field (SDF), NeRF-based approaches can perform 3D reconstruction learning an accurate continuous representation of the volume.
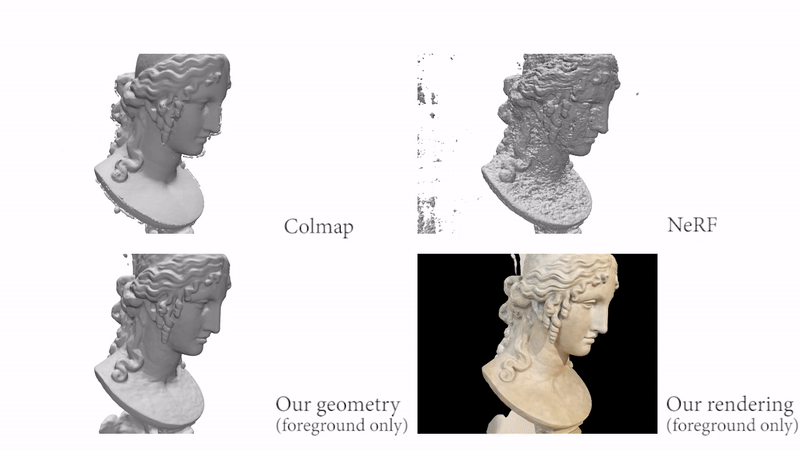
Key research topics include:
- 3D INDOOR RECONSTRUCTION: indoor scenes are generally more challenging due to their size and the high amount of details. We research solutions to produce reconstructions with a reliable geometry, exploiting external geometrical priors such as multi-view stereo depth maps and SfM point clouds.
- MULTI-DOMAIN NEURAL RADIANCE FIELD: we are interest in the possibilities offered by data belonging to domains different from standard RGB. The idea is to exploit alternative solutions to improve the reconstructed geometry and to define new approaches for the material property estimation. The model must be able to return a complete asset, easy to import in the popular software for modelling and rendering.
Recent publications:
Exploiting Multiple Priors for Neural 3D Indoor Reconstruction Proceedings Article
In: 34th British Machine Vision Conference, 2023.