More recently, compression strategies have been adopting deep learning architecture to achieve high compression ratios while granting a pleasant and satisfying visual quality. In our investigation we focus on feature optimization for autoencoder-based codecs, generative coding strategies based on diffusion models, coding schemes based on neural implicit representation (NIR).
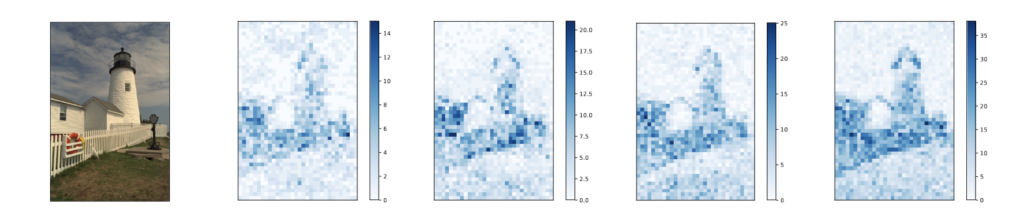
Key research topics include:
- image/video/audio/depth/ligh-field compression
- learned image compression
- coding scheme based on diffusion models
- coding schemes based on neural implicit representations (NIRs)
- image/video/audio/ligh-field rendering based on deep learning architectures
Selected publications:
CACTUS: Content-Aware Compression and Transmission Using Semantics for Automotive LiDAR Data Journal Article
In: Sensors, vol. 23, iss. 12, 2023.
Recent advancements in learning algorithms for point clouds: An updated overview Journal Article
In: Sensors, vol. 22, no. 4, pp. 1357, 2022.
Adae: Adversarial distributed source autoencoder for point cloud compression Proceedings Article
In: 2021 IEEE International Conference on Image Processing (ICIP), pp. 3078–3082, IEEE 2021.
A syndrome-based autoencoder for point cloud geometry compression Proceedings Article
In: 2020 IEEE International Conference on Image Processing (ICIP), pp. 2686–2690, IEEE 2020.
A transform coding strategy for dynamic point clouds Journal Article
In: IEEE Transactions on Image Processing, vol. 29, pp. 8213–8225, 2020.