During the last years, we all developed a growing dependency on communication services. In turn, the number of connected devices is increasing and the security threat scenario is constantly evolving. This creates the need for new and effective security solutions. For this reason, the definition of new approaches for the detection of network anomalies has become a key research topic to investigate on.
But what is an anomaly in the first place?!
An anomaly can be defined as an unusual behavior of a system. This could be due to malicious actions or to genuine but atypical ones. A legitimate user with no intention to compromise the network security, in fact, could cause an unusual network behavior.
Due to its complex nature, anomaly detection and the distinction between malicious and genuine anomalies is a challenging task.
Concerning anomaly detection techniques, they can be split in two groups: signature-based and profile-based. The first category tries to detect known anomalies thanks to a prior definition of their features. Profile-based approaches, on the contrary, exploit the history of the normal network behavior for creating a normal network profile. Following this principle, we consider an “anomaly” as a network behavior that is significantly different from the modeled one.
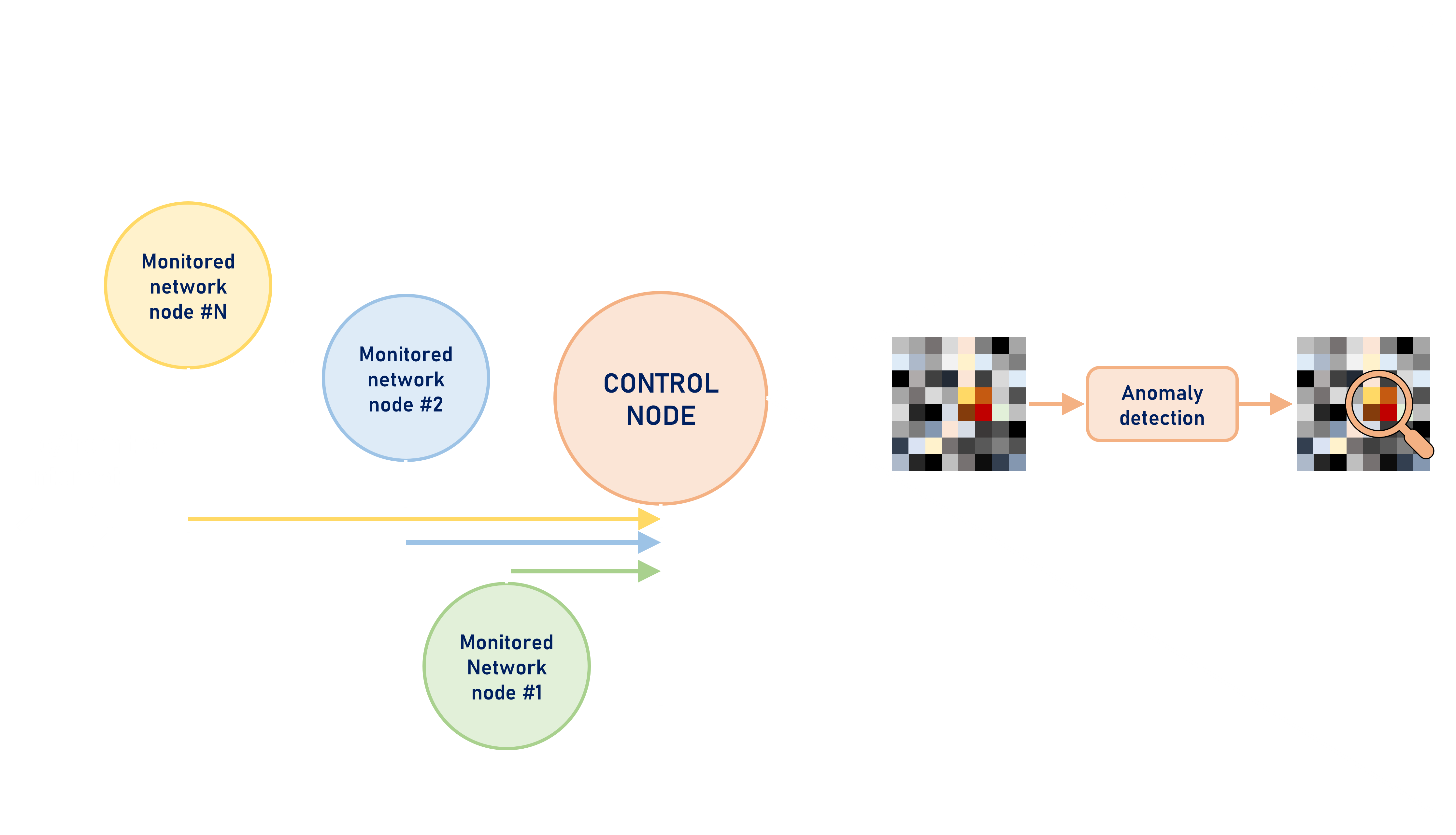
Key research areas include:
- Definition of an effective two-dimensional representation of network traffic which allows to characterize the traffic of the monitored network in a compact form.
- Design and implementation of a profile-based network anomaly detection system based on the processing of the 2D traffic representation which exploits techniques usually employed for images.
These activities are carried out in the context of the ISEEYOO project. If you are curious, you can find out more in the “Projects” page.
Recent publications:
Histogram-based network traffic representation for anomaly detection through PCA Journal Article
In: Computer Networks, pp. 111276, 2025, ISSN: 1389-1286.
Analysis of a 2D Representation for CPS Anomaly Detection in a Context-Based Security Framework Journal Article
In: Frontiers in Signal Processing, vol. 1, pp. 19, 2022.
Unsupervised Network Anomaly Detection by Learning on 2D Data Representations Proceedings Article
In: 2022 9th Swiss Conference on Data Science (SDS), pp. 53–58, IEEE 2022.